Four steps to bring success to your Machine Learning project
When considering Machine Learning as a part of your company’s IT toolbox, there are certain things that are pivotal to address. We will touch upon how your approach to ML projects may impact the outcome.
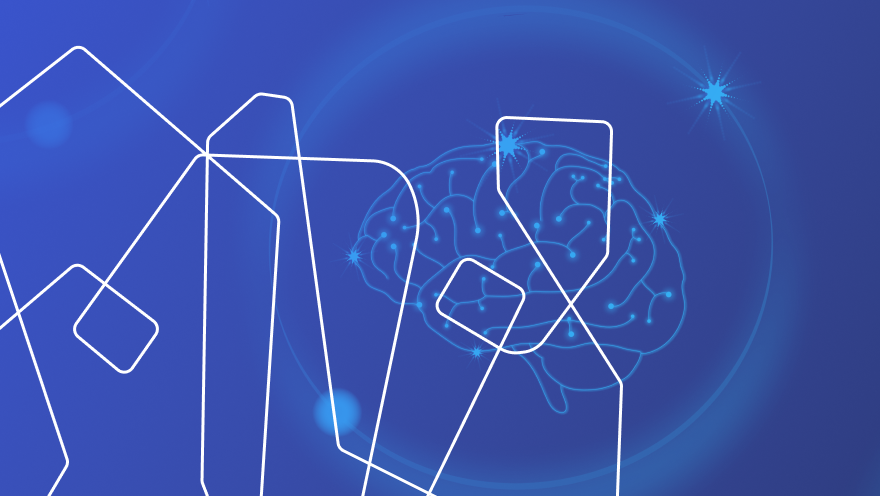
"Where can AI and ML be applied?" is a common question among business owners. The answer is: Everywhere – with a proper approach. Machine Learning works wonders predicting expensive incidents and unexpected expenditures, prioritizing and processing customer requests, detecting anomalies, for pattern recognition, intelligent text analysis and recommendation systems.
Based on the real-world cases – a system for analyzing ultrasound scans in medical diagnosis, a vehicles’ route prediction system, an inbound lead ranking system for air ticket sales, a shopping app defining user preferences, a module to detect users’ fraudulent activity, to name a few – WaveAccess has developed an approach that helps smoothly integrate ML in business processes. With respect to any business area and regardless of the company’s size.
Step #1 – Data collection, processing and verification
The starting point is the relevant data. When it comes to Machine Learning, it is much more difficult to formalize the processes, specify the terms and evaluate the required resources before obtaining the data. Data shortage or incomplete data, missing or unreliable labeling and incorrect data may lead to a less than complete view of the problem. Remained unaddressed, it will quite simply result in a model lacking accuracy – which is a less than effective solution.
Those who prepare well are more likely to succeed. Get all the data and get it in order, make sure you know where it comes from, and objectively assess its quality – be honest with yourself and your development partner. If you accept substandard data, the outcome will be substandard regardless of the ML algorithm. And don’t panic about data security – anonymized data also works.
But let’s be real, the ideal basis – realistic, correct and properly labeled data in the right quantity – is almost never the case. Luckily, there are proven ways to deal with it.
Step #2 – Prototyping
When developing something as strategic and complex as Machine Learning models you have to make sure that they will behave as expected. The only way to secure that is to create a prototype and test it prior to the final development and implementation.
There is every reason to invest in prototyping. It helps understand what accuracy rate the ML model can provide on the available dataset, and what optional resources might be required to improve it. Therefore, prototyping is pivotal to ensure a Machine Learning project runs smoothly and to avoid errors that might result in having an incorrect foundation for decision making and optimization.
After all, you don’t just build an airplane, welcome people on board and then hope it will fly safely, do you?
Step #3 – Expectation management and ROI
Expectation management is crucial. Always make sure that processes, time frames, estimated investment and expected outcome are agreed on. Be certain you have the ROI model calculated beforehand – it will show what efficiency really makes sense for investments to pay off and whether it’s worth investing in improving the model. A 100% accuracy rate looks attractive but can often be unjustified. Also note that once the solution is ready, you can still step back and estimate whether it is possible to improve the model and how much it will cost.
Step #4 – Flexible approach to planning
Each Machine Learning project requires R&D, as it is not an off-the-shelf solution. Such projects are dynamic, they can change course based on data provided, resources allocated, changing ambitions and desired outcome, and therefore may also require additional costs and efforts. Be honest and upfront about what you expect, as what you hope for might not always be realistic.
Which brings us back to the very first question that should be addressed: Is Machine Learning really required as the best solution to the problem at hand?
With 19 years of experience in software development, over 5 years of experience in ML and AI project development and a portfolio of 20+ related projects, we have obtained quite a vast amount of insights. ML is not a universal remedy: tons of problems can be solved quicker and cheaper, and there is no point in investing in technology just for the sake of it.
Though these tips and tricks are simple when exploring them on paper – they are determinant once your project starts. We therefore urge you to take them seriously and use this checklist as a foundation when searching for a tech partner. We dare to say that if your potential partner does not stick to them, they might not have your best interest at heart.
Let us tell you more about our projects!
Сontact us:
hello@wave-access.com