Building a solid foundation for AI success in treasury
AI only delivers value when built on a strong foundation. Most treasury teams aren’t held back by AI itself — but by fragmented data, disconnected systems, outdated processes, and lack of visibility. Explore a practical approach to getting your organization AI-ready.
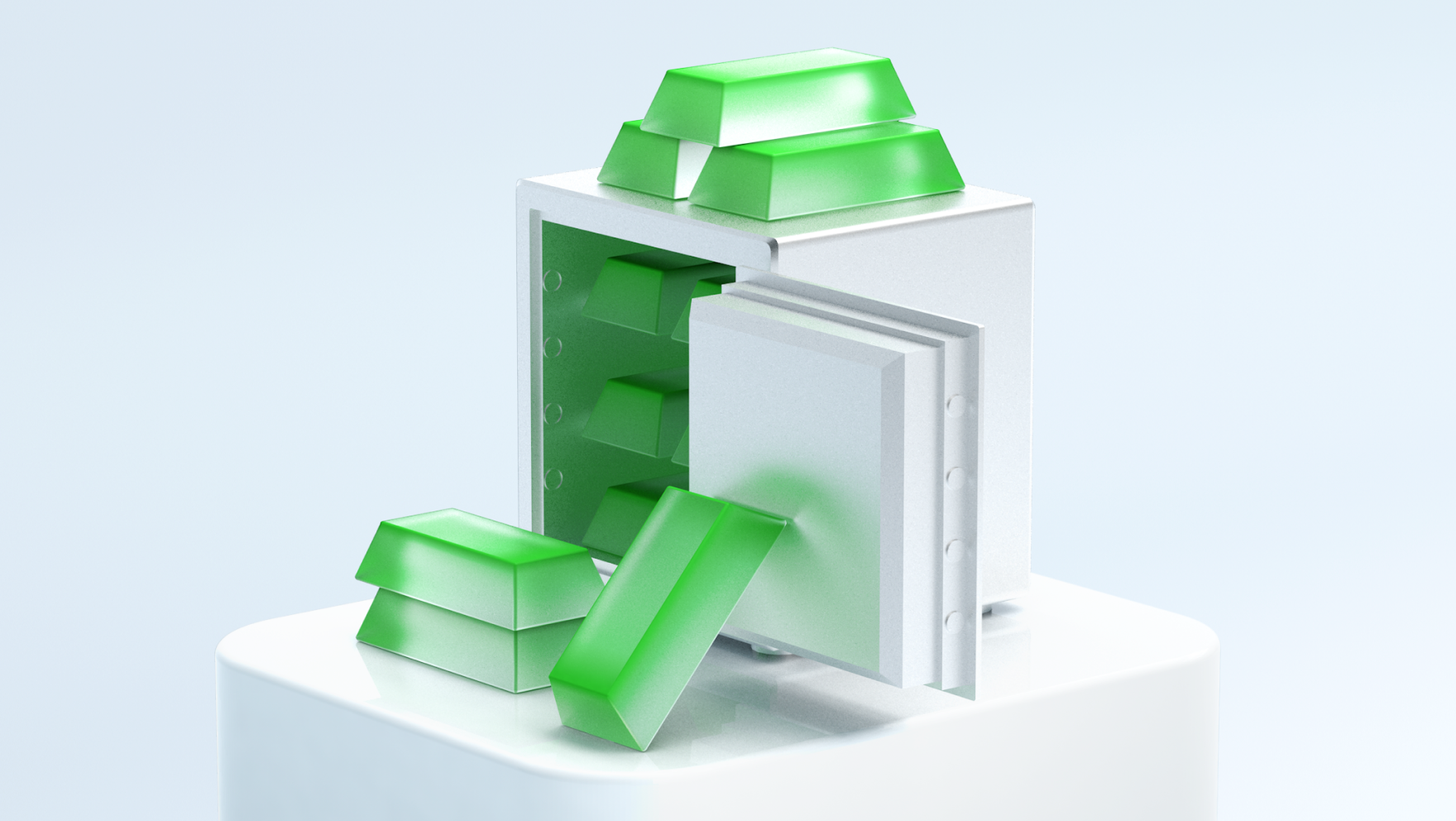
AI doesn’t drive results on its own. It needs the right environment and foundation to deliver real value. And preparation starts long before you deploy any AI tool. Let us walk you through a number of practical steps your organization needs to take to prepare for sustainable AI adoption.
The layered approach to AI preparation
We suggest to follow the layered approach — it’s a simple but powerful way to think about preparing your treasury for AI.
It all starts with your data layer. This is everything you work with daily: internal data from your ERP or TMS, external data like bank statements or market feeds — all stored and organized in your internal systems.
Once that’s in place, you move to the data integration and processing layer. This is where you connect those different data sources and set up automated processes to keep your data accurate and up to date. For example, triggering notifications when new payments come in or when data changes require attention.
Next comes the data visualization and business flows layer. This is where your team can finally see what’s going on — with dashboards for cash visibility, payment tracking, or forecasting, and tools that support your daily workflows.
And finally — the outer layer is where AI comes in. This is where machine learning and Large Language Models help you analyze your data, surface insights, and speed up decision-making.
Each layer builds on the previous one. If the foundation isn’t solid — AI won’t deliver the results you expect.
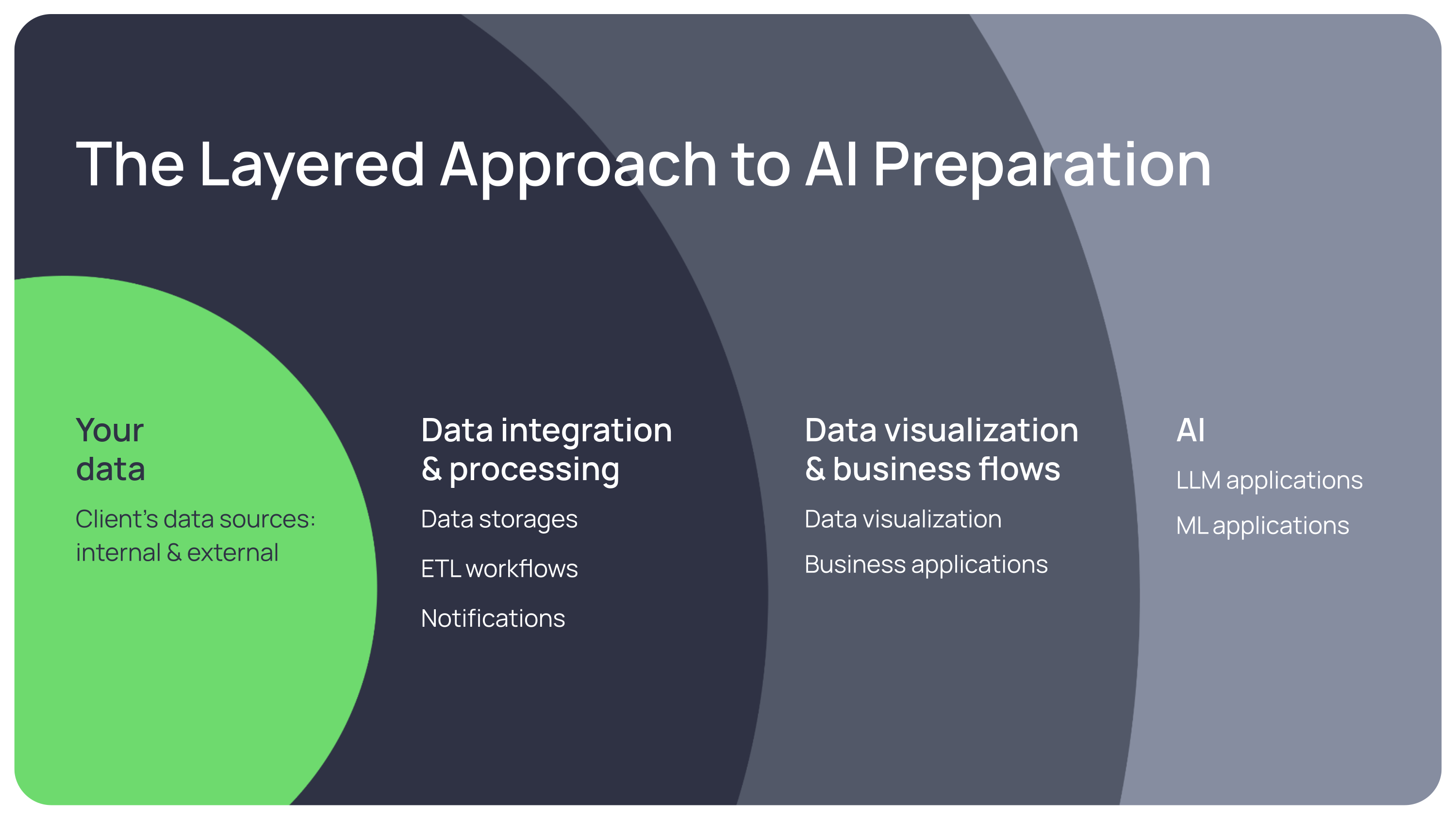
Layer 1: Get your data house in order
The key questions here are simple: Can you trust your data? Is it accurate, complete, and consistent across systems?
The harsh reality is — if your data isn’t clean, no AI tool is going to fix that. It’ll just give you wrong answers faster.
Let's see a couple of real-life treasury examples.
Case 1: Inaccurate cash flow forecast from bad AP data
A Treasury Manager was building a 13-week cash flow forecast. They pulled a report from the ERP — but it turned out that several supplier payments were listed twice.
As a result, the forecast showed $1.5 million more in outflows than reality.
What happened next?
- The team expected a liquidity shortfall and drew on a line of credit unnecessarily.
- This led to added interest expense, and they missed an opportunity to invest idle cash.
- It also damaged confidence in the treasury's reporting among senior leadership.
The lesson is clear: If your AP data isn’t clean and validated — your forecasts are useless, and can result in poor financial decisions.
Case 2: Missed discounts and late fees due to disorganized payment terms
A Treasury Director was trying to optimize working capital, but quickly ran into a problem. Supplier payment terms were all over the place: some in ERP, some in emails, some buried in PDF contracts.
What was the impact?
- The company missed out on early payment discounts from key suppliers.
- In other cases, payments were made too late, incurring late fees and damaging vendor relationships.
- Working capital metrics like Days Payable Outstanding (DPO) became impossible to benchmark or manage.
The lesson here is that if your payment terms aren’t centralized and searchable, you can’t optimize AP — with or without AI.
Steps to assess and prepare your data for AI
What can you do to get your data ready? Here’s a simple checklist to start with:
-
Run a data health check first
AI will only work with clean, organized data. If your data is flawed, even AI-driven insights will be misleading. So, look for duplicates, missing fields, outdated records.
-
Centralize and standardize key data
Dispersed or inconsistent data leads to confusion and errors — standardization allows AI to work effectively and ensures it pulls reliable insights.
Store critical information — like payment terms, customer aging, or cash forecasts — in one consistent place.
-
Make sure you clean your data and validate it regularly
Regular data cleaning ensures your forecasts are accurate and that you can rely on data for strategic decision-making.
Set up regular routines to deduplicate and update data.
-
Align data with your treasury needs
Misaligned data can lead to wrong AI outputs. Ensure the data you gather serves the specific needs of the treasury function.
Structure your data around what your team needs — for forecasting, AR, payments — not just what’s easy to collect.
Layer 2: Integrate systems and automate data flows
Once your data is clean and reliable, the next questions are: Can you actually use it easily? Can your treasury team quickly pull together data from all key sources? Is that data always up to date and ready for analysis?
For many treasury teams, the answer is still no, and that’s a major roadblock on the way to AI adoption.
Picture this: a Treasury Manager wants to check cash position — but invoice data sits in the ERP, bank data lives elsewhere, and contract details are buried in emails. Or take a Treasury Analyst reconciling payments — they might spend hours manually matching records because bank payments and ERP invoices don’t “talk” to each other.
These kinds of manual workarounds slow you down — and they make it nearly impossible for AI to deliver real-time, reliable insights.
The good news is that this is fixable. The goal at Layer 2 is to connect your data sources — so information flows automatically between systems. No more hunting through spreadsheets or emails, no more manual updates.
This is the stage where you work closely with your IT team to link ERP, bank, and contract data, automate updates, and build a unified data flow treasury can rely on.
This sets the stage for what comes next: clear, real-time visualization and process automation — which we’ll cover in Layer 3.
Case: The email trap in procure-to-pay
When treasury teams think about data, they usually focus on ERP systems, banking platforms, and payment tools. But if you want to truly optimize working capital — and prepare for AI — you need to go deeper.
Specifically — into procurement, as it holds a lot of critical information that treasury teams need to plan cash outflows properly. These are real-time order confirmations, delivery status updates, negotiated payment terms, approved (but not yet invoiced) commitments, and early payment discounts or penalties.
But what happens in many companies is that this information is locked in emails, or stuck in spreadsheets, or sitting in procurement tools that don’t connect to ERP.
And that’s exactly what happened in the following case. The treasury team wanted better cash flow visibility and smoother vendor payments. But procurement was sending order confirmations and delivery updates by email directly to AP. None of it was stored in a structured way.
Payment terms were changing, delivery dates were shifting — but all those updates were buried in email threads, and nothing was reflected in ERP.
As a result:
- Treasury couldn’t see which POs were confirmed
- They didn’t know when deliveries were expected
- Their cash flow forecasts were constantly off
The AP team was left manually forwarding emails, copying data into spreadsheets, and reconciling purchase orders by hand — every single week.
To fix this, an integration layer was built to connect Outlook directly to their contract management system — allowing teams to file emails with a single click. Then, ERP and contract data were connected — so the treasury team could see real-time payment obligations and approval statuses automatically.
The impact?
- Treasury gained visibility into committed spend before it hit accounts payable
- Forecasts became more accurate
- Cash buffers were easier to manage
- And AI could finally help them spot risks — like when discount terms were about to be missed
But none of that would’ve been possible without reaching beyond ERP and pulling procurement data into the picture.
Steps to build a data integration and processing foundation
Here are four steps to turn fragmented, manual treasury operations into a connected, real-time data ecosystem — ready for automation, reporting, and AI:
-
Connect your data systems
Make sure all your key data sources — procurement, AP, contracts, ERP, banking — are connected. If these systems don’t talk to each other, you’ll always have blind spots. And those blind spots create risks and errors.
-
Standardize data formats
Even if your systems are connected, your data still needs to speak the same language. Implement consistent formats for things like payment terms, vendor names, or contract details. The cleaner and more consistent your data, the easier it is to process — and the more accurate your AI insights will be later.
-
Automate data transfers
Manual data entry slows you down and opens the door for mistakes. Wherever possible, replace manual processes with automated data flows between systems. This not only saves time — it ensures your data is always current and ready for analysis.
-
Maintain data integrity
Finally, integration isn’t a “set it and forget it” exercise. Make data cleaning and validation part of your regular process. Accurate, up-to-date data is what gives you reliable forecasts, better reporting — and makes sure your future AI tools actually deliver value.
Layer 3: Build dashboards to enable data visualization
This is where we shift from just having data to actually using it in real time.
At this point, ask yourself: Can your treasury team see key data clearly — like cash positions, forecasts, or payment statuses? Are your processes — like reporting or forecasting — built to react fast when things change?
And for many treasury teams, this is exactly where things start to fall apart. You’ve probably seen it yourself — reporting built on outdated snapshots, forecasts that take so long to prepare they’re already out of date by the time they’re ready.
Picture this: a Treasury Director is getting ready for a board meeting. They’re looking at reports pulled a week ago — completely unaware that a major payment just landed yesterday. That one missed detail could change the whole liquidity picture, but it never even shows up on their radar.
Or take a Treasury Manager building a cash flow forecast by manually collecting data from different sources, cleaning it, double-checking it. Hours go by. And by the time they hit “Send” — something in the market has already shifted.
This is exactly why Layer 3 is so important. You need to give your team real-time visibility into your key metrics — and automate the manual stuff wherever possible.
- Dashboards that show cash positions or payment statuses in real time.
- Automated reporting that updates without anyone touching a spreadsheet.
- Processes designed for speed, not busywork.
Only when your team can see what’s happening and act on it quickly — you get truly ready to add AI on top.
Case: Simplifying to drive adoption
Here’s something we see quite often when teams move to dashboards and real-time reporting — and it’s a mistake that can quietly kill adoption.
It usually starts with good intentions. The team wants to build dashboards that show everything: exchange rates, regional balances, payment queues, investment schedules — all packed into a single screen. And it looks impressive at first glance.
But in practice it quickly becomes overwhelming. No one knows where to look, the dashboards turn into cluttered information walls — and people quietly go back to Excel because it’s faster and easier.
The fix here is simple, but powerful: one screen, one purpose. Every dashboard should answer a specific question — like “Can I invest this week?” or “Are we on track with our DPO target?”
Steps to enable data visualization and streamline business flows
If you want dashboards to work for treasury — focus on clarity, speed, and usability.
-
Keep dashboards simple
Every dashboard should have a clear purpose. Not “show me everything at once” — but a focused question like: “Can I invest this week?” or “Where are we with collections?” That focus helps users make faster, more confident decisions.
-
Limit data overload
It’s tempting to fill dashboards with every possible metric — but that only slows people down. If users have to scan 20 charts to find what they need, they’ll stop using the tool altogether. Keep it clean, clear, and relevant.
-
Train your team
This is often overlooked. Don’t assume people will figure it out on their own. Offer role-based training — show treasury managers, CFOs, AP teams exactly how to use the dashboards in their daily work. Adoption goes up dramatically when people know exactly what to do.
-
Automate key processes
Dashboards work best when they’re fed by real-time, automated data. Automate forecasting, reporting, any repetitive task that takes time away from strategic work. That’s what allows your team to focus on what really matters — making decisions, not managing spreadsheets.
Layer 4: Introduce AI to treasury operations
At this point, with clean data, integrated systems, and automated processes in place — you’re finally in a position to get real value from AI.
AI isn’t here to replace treasury expertise — it’s here to support it. To help teams work smarter, move faster, and focus on higher-value decisions.
What can AI actually do at this stage?
- Help you analyze large amounts of data in seconds.
- Surface patterns or risks you might miss.
- Retrieve critical information instantly.
- Enable faster, more informed decision-making across treasury functions.
AI Assistant: Analytics and Search
AI Assistants introduce a practical shift in how treasury teams work with data and make decisions. Think of them as on-demand analysts who understand natural-language questions, know where to look across systems, and explain financial scenarios in straightforward terms.
Powered by large language models (LLMs), these assistants are especially effective at interpreting context, retrieving the right data, and delivering clear responses. They still rely on predefined rules for calculations and financial outputs, but their value comes from combining contextual understanding with smart backend integrations.
For treasury professionals, this enables two key capabilities:
-
Business-aligned data analysis
Goes beyond presenting figures by connecting them to real business questions — such as showing how an interest rate shift would affect your debt profile.
-
Context-aware data search
Quickly surfaces relevant information from multiple systems — whether you’re looking for overdue invoices, regional exposures, or counterparty risk assessments.
This results in faster answers, better visibility, and fewer manual steps — assuming the right data foundation is in place.
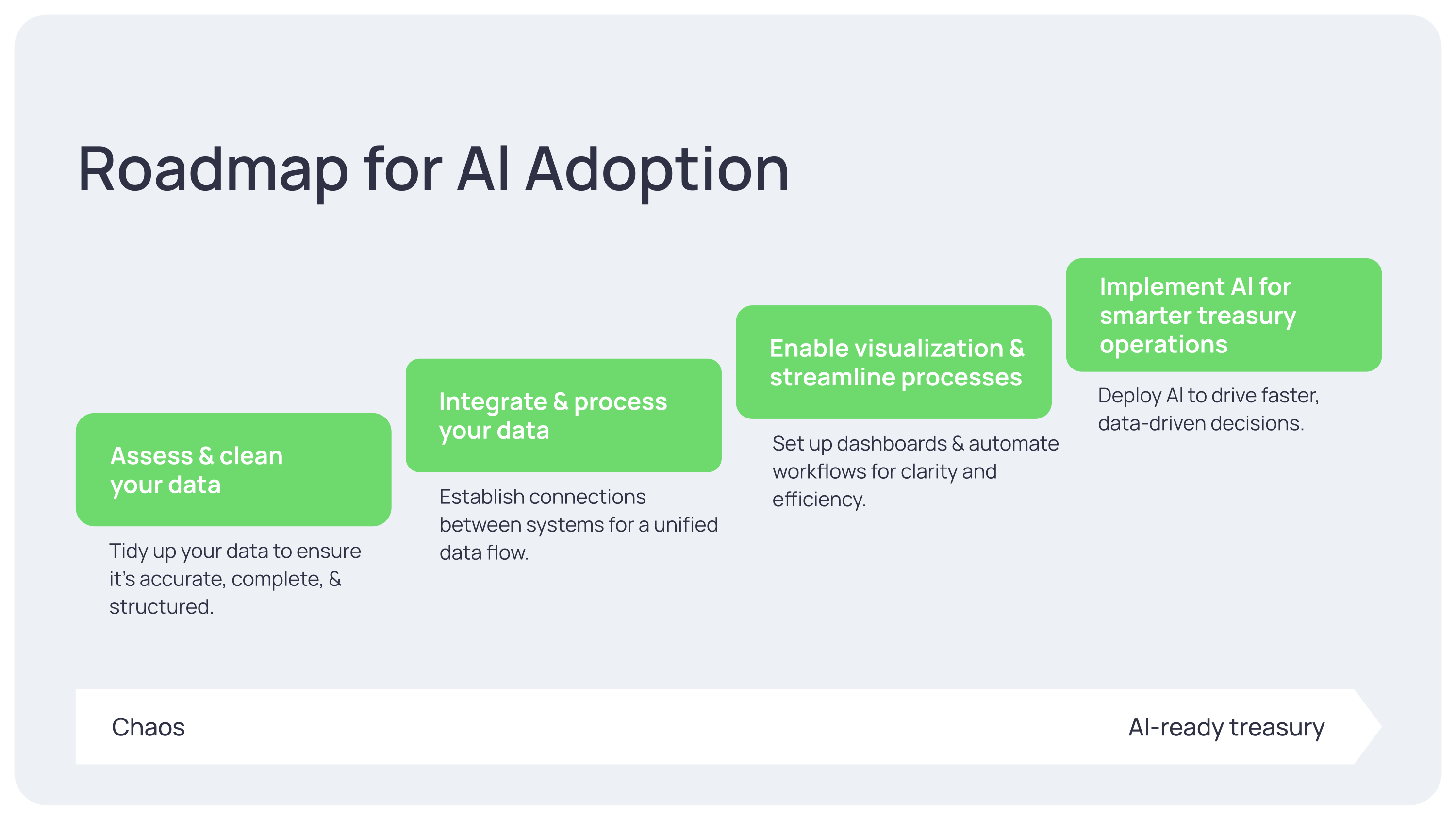
Your roadmap for AI adoption
Adopting AI in the treasury isn’t something you can do overnight. It’s a process — and like any process, success starts with building the right foundation. This is your path from preparation to real AI-driven transformation — and it follows the exact structure we’ve discussed.
-
It all starts with a data health check.
-
Next, it’s about connecting your key systems — ERP, banks, contracts — and making sure data flows automatically between them. The outcome would be — a single, unified data flow that’s always up to date and ready for analysis.
-
Then, you make that data easy to use. Set up dashboards, automate reporting, and eliminate manual, repetitive tasks wherever you can.
-
Finally — once your foundation is in place — that’s when it makes sense to bring in AI. Start small: pick a very specific use case — like improving cash flow forecasting or spotting payment risks early — and build from there.
Moving through these steps isn’t just about technology. It’s about collaboration across the business: treasury defines what success looks like, IT connects the systems, finance ops ensures the data is clean and reliable, and leadership drives alignment and buy-in. And along the way, it’s crucial to build the right skills — data literacy, AI literacy, and change management.
Because at the end of the day — successful AI adoption happens when people, processes, and technology come together in the right way.
In the next part, we'll show how treasury AI Assistants work in practice — from daily operations to strategic planning.
Let us tell you more about our projects!
Сontact us:
hello@wave-access.com